Why do data scientists waste up to 70% of their time and money collecting and cleaning data?
12 April 2022
The following article will focus on understanding, highlighting, and sharing some insights on the real reasoning behind why do data scientists waste valuable time collecting and cleaning their data. The article will also briefly delve into the real “cost” of managing high quality data.
Since 2008 we have been working with different customers worldwide easing their trading process, we now would like to answer the long-debated question of “Why do data scientists waste up to 70% of their time and money collecting and cleaning data?”
Upon extensive research we have concluded that there are an array of factors contributing to this reason. These particular factors are stated as follows;
Firstly, we were made aware that the data format is not necessarily user friendly or adapted for the end user’s usage, hence making it harder to navigate through and subsequently leading to longer data sourcing hours. In fact, the data delivered by the providers to the users is much more a storage data than a processing data.
One of the data providers main concerns is optimizing storage space (hundreds of tera bytes a day). Besides, almost all data providers record the data coming from different exchanges with a full subscription to widen the coverage (subscribe to all the assets of a given exchange) rather than using a client watch list. Sometimes, they have to split the full order book, the trades and the reference data into different archives files.
Additionally, data is recorded as tick by tick. In an environment where every tick counts, this can be lethal and has detrimental consequences on one’s trading process, due to the mere fact that it is not working at the optimal pace and as such clients aren’t able to base and compare their trading decisions with the most up to date data making it somewhat unusable. The time stampings need to be adjusted with the UTC time zone and the exchange local time zone. Moreover, not all the exchanges or the providers are using the same symbology; we need to map the data using different codes.
Advancing on to the topic of the incurred cost which comes with managing high quality data, we have summarized that the cost of transferring data across networks is often highly costly. Another factor contributing to the elevated costs is the fact that one is required to download substantially more data every day. Alongside of this, storing data is also classified as a costly activity.
Case study
A recent client highlights these issues: our client is aiming to back-test a new trading strategy on the CME futures. As an input, he needs to build 5 minutes bars based on the tick-by-tick data (trades and top of the book) with consideration for the trading conditions (remove the OTC or block trades for example).
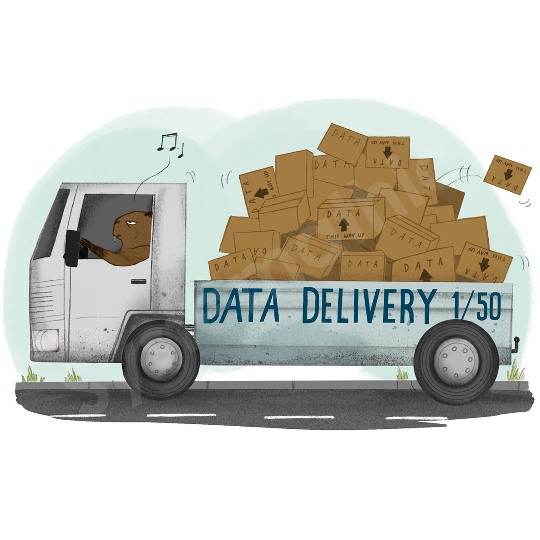
Below are the costs in terms of storage (S), download (D) and processing (P). The data is provided by one of the top three market data providers:
Duration | FTP data (6 sources) Tick by Tick | Watch list only (25 assets) Tick by Tick | Watch list only (25 assets) Sampled data (5 min bars) |
---|---|---|---|
1 Day | S, D : 1 GB (GZIP) P : 5 GB (ASCII) |
S : 80 MB P : 425 MB |
P : 1 MB |
1 Year | S, D : 250 GB (GZIP) P : 1.25 TB (ASCII) |
S : 20 GB P : 100 GB |
P : 250 MB |
5 Years | S, D : 1.25 TB (GZIP) P : 6.25 TB (ASCII) |
S : 100 GB P : 500 GB |
P : 1.25 GB |
90% unused data | 99% unused data |
To achieve our very basic scenario:
- We downloaded 1.25 TB of data: the provider’s FTP bandwidth is limited to 100mbits/sec: +2 days of 24/24 DL
- Stored 1.25 TB on local device: 1 HDD is required (more than 100 DVDs)
- Needed to process 6.25 TB plain ASCII data: days of CPU processing
Only 1.25 GB of data is useful and the whole back-test runs in less than 5 seconds!
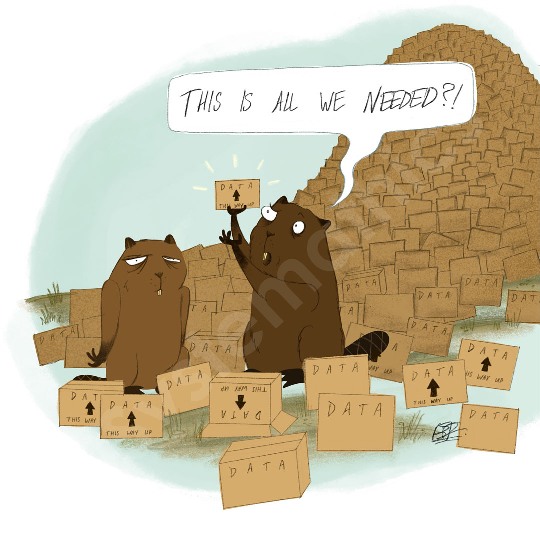
Conclusion
While there is no exhaustive list in this article to explain the reasons of why do data scientists are spending a major part of their time in data wrangling, the result of not having good data processing system is costly for both data scientist and the team.
We need to free data scientists to put their skills to best use.